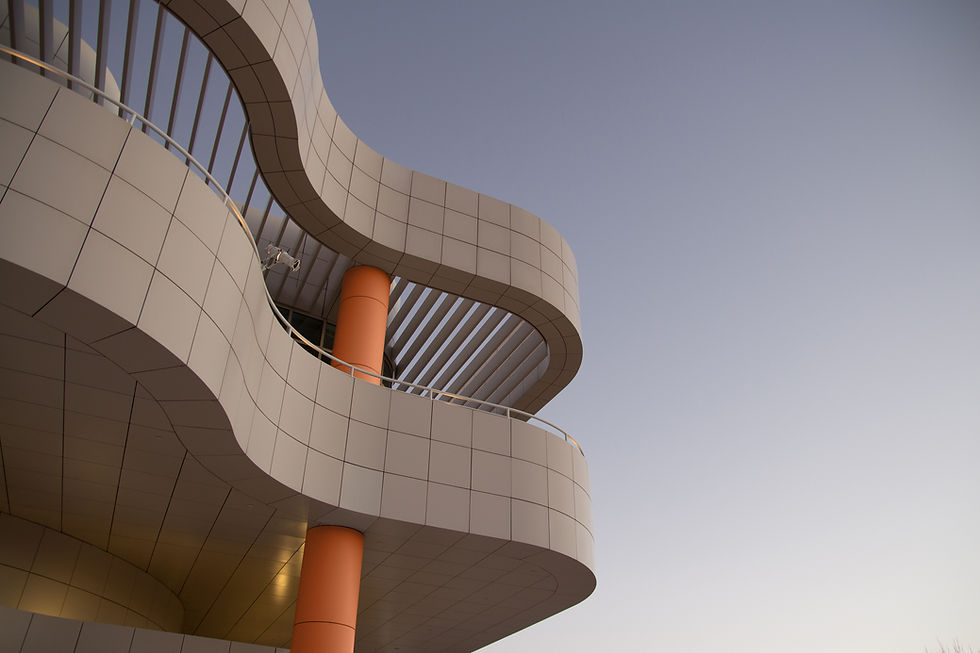
The Exponential Growth of AI Technology: A Comparison with Blockchain Advancements
Nov 8, 2024
6 min read
0
4
Introduction
In an era marked by rapid technological progress, the exponential growth of AI technology stands out as a transformative global force. This growth is not just accelerating; it's reshaping industries, economies, and societies as we know them. From the optimization of complex operations to the creation of innovative products and solutions like ChatGPT and generative AI, the capabilities and potential of artificial intelligence are expanding at an unprecedented pace. Understanding the significance of this exponential AI expansion, and how it compares with other technological advancements such as blockchain, is crucial for grasping the future trajectory of global innovation.
This article offers a deep dive into the phenomenal growth of AI technology, providing an overview of its evolutionary journey and the factors driving its rapid advancement. A comparative analysis with blockchain technology's growth will serve as a benchmark, illustrating the unique pace and impact of AI's development. By exploring the future outlook for AI and reflecting on its implications across various sectors, this piece aims to provide readers with a comprehensive understanding of the dynamism characterizing today's technological landscape.

Overview of AI Technology Growth
Key Milestones in AI Development
The journey of AI technology over the last decade is marked by significant milestones that have shaped its current capabilities. Generative Adversarial Networks (GANs), introduced in the early 2010s, revolutionized the creation of realistic AI-generated images and videos. The launch of Google DeepMind's WaveNet in 2016 transformed the generation of natural-sounding computer voices, moving away from the robotic tones produced by earlier technologies. That same year, AI achieved a historic milestone when DeepMind's AlphaGo defeated the world champion at Go, a complex board game long considered a benchmark for AI prowess. More recently, in 2020, the AI algorithm AlphaFold made significant strides in predicting protein structures, a critical task in biological research.
The rapid adoption of OpenAI's ChatGPT following its 2022 release underscores the growing influence and capabilities of AI, as it became the fastest-growing consumer application in history. From this point, the race in generative AI models truly began, with an unprecedented acceleration in the development and release of new technologies.
OpenAI Timeline and Release Frequency
GPT-1: June 2018
GPT-2: February 2019 (~8 months after GPT-1)
GPT-3: June 2020 (~16 months after GPT-2)
Codex: August 2021 (~14 months after GPT-3)
GPT-3.5: March 2022 (~7 months after Codex)
ChatGPT: November 2022 (~8 months after GPT-3.5)
GPT-4: March 2023 (~4 months after ChatGPT)
GPT-4 Turbo: November 2023 (~8 months after GPT-4)
GPT-4o: May 2024 (~6 months after GPT-4 Turbo)
GPT-4o Mini: July 2024 (~2 months after GPT-4o)
This timeline clearly illustrates the accelerating pace of AI development. What started as yearly or multi-year gaps between major releases has now compressed to mere months. The interval between GPT-4o and GPT-4o Mini, at just two months, is particularly striking and demonstrates the breakneck speed at which AI technology is evolving.
OpenAI's rapid release cycle has sparked intense competition among tech giants and startups alike, leading to a flurry of new AI model releases and improvements across the industry. This pace has forced other companies to accelerate their own AI development efforts, resulting in a highly competitive and fast-moving landscape. Google's response with Bard, Anthropic's Claude, and Meta's LLaMA are just a few examples of how quickly the field has expanded.
Anthropic's Release Cycle
Claude 1: March 2021
Claude 2: July 2023 (~28 months after Claude 1)
Claude Instant 1.2: November 2023 (~4 months after Claude 2)
Claude 3 Family: March 2024 (~4 months after Claude Instant 1.2)
Claude 3.5 Sonnet: June 2024 (~3 months after Claude 3 Family)
Claude 3.5 Haiku and Opus: Expected late 2024 (~6 months after Claude 3.5 Sonnet)
Anthropic's rapid release cycle suggests they're actively working to keep pace with or surpass competitors like OpenAI. This rapid progression has not only pushed the boundaries of what's possible with AI but has also sparked important discussions about AI ethics, regulation, and the potential societal impacts of these powerful technologies. The speed of releases and improvements in AI capabilities continues to outpace many predictions, highlighting the dynamic and transformative nature of this field.
Factors Driving AI Growth
The exponential growth of AI is fueled by several key factors:
Availability of Large Datasets: The abundance of data from various sources, including social media, sensors, and enterprise systems, has provided the raw material for training sophisticated AI models.
Advanced Computing Power: The development of powerful GPUs and specialized AI hardware, such as TPUs, has significantly increased the computational capacity available for AI research and deployment.
Innovations in Hardware and Software: Continuous advancements in both hardware (e.g., quantum computing) and software (e.g., optimized algorithms) have driven AI forward.
Demand for Automation and Intelligent Decision-Making: Businesses and consumers alike are increasingly seeking automated solutions and intelligent decision-support systems.
Investment in Infrastructure: Major investments by tech giants in AI infrastructure have been critical, providing the resources necessary for large-scale AI research and development.
Cost of Energy: The lowering cost of energy has made it more economically feasible to operate the large data centers required for AI processing.
Impact of AI Across Industries
AI's impact is evident across a wide array of sectors, transforming operations and driving innovation:
Healthcare: AI applications are revolutionizing medical diagnoses and treatment development. AI algorithms analyze vast amounts of medical data to identify patterns and predict outcomes, improving patient care and operational efficiency.
Finance: The finance sector has embraced AI for automated and precise decision-making processes, from fraud detection to personalized financial advice.
Manufacturing: AI-driven automation enhances efficiency and productivity in manufacturing, reducing the prevalence of human error and enabling predictive maintenance.
Legal and Marketing: In the legal sector, AI automates tasks like document review, while in marketing, AI personalizes strategies and optimizes campaigns for better engagement.
Non-Profit Organizations: AI aids in decision-making and resource allocation, maximizing the impact of non-profit efforts.
Comparing AI Growth with Blockchain Advancements
While AI has been rapidly evolving, blockchain technology has also seen significant growth, though at a different pace and scale. Blockchain, known for its decentralized ledger system, has found applications in finance, supply chain management, and digital identity verification.
Key Milestones in Blockchain Development
Blockchain technology gained prominence with the introduction of Bitcoin in 2009, followed by the emergence of Ethereum in 2015, which brought smart contracts to the forefront. These smart contracts have enabled automated, secure, and transparent transactions, paving the way for decentralized applications (dApps).
Blockchain Timeline and Release Frequency
Bitcoin: 2009
Ethereum: 2015 (~6 years after Bitcoin)
Hyperledger Fabric: 2016 (~1 year after Ethereum)
EOS: 2018 (~2 years after Hyperledger Fabric)
Polkadot: 2020 (~2 years after EOS)
Cardano: 2021 (~1 year after Polkadot)
Unlike AI, blockchain technology has seen more measured and deliberate development, focusing on establishing a robust and secure infrastructure. The release of new blockchain platforms and improvements has typically followed a slower, more iterative process compared to the rapid iterations seen in AI.
Factors Driving Blockchain Growth
The growth of blockchain technology is driven by several factors:
Decentralization and Security: Blockchain's inherent security and decentralized nature have made it attractive for various applications, particularly in finance.
Transparency and Trust: The immutable nature of blockchain ledgers provides transparency and trust in transactions, crucial for sectors like supply chain and digital identity.
Regulatory Environment: The evolving regulatory landscape has influenced blockchain's adoption and development, with regulations providing both opportunities and challenges.
Interoperability and Scalability: Continuous efforts to improve blockchain interoperability and scalability have been essential for its broader adoption.
Impact of Blockchain Across Industries
Blockchain technology has significantly impacted several industries:
Finance: Blockchain has revolutionized finance through cryptocurrencies, smart contracts, and decentralized finance (DeFi) platforms, enabling secure and efficient transactions.
Supply Chain Management: Blockchain enhances supply chain transparency and efficiency by providing an immutable record of transactions and movements.
Healthcare: Blockchain's secure data handling capabilities are being leveraged for patient data management and pharmaceutical supply chains.
Digital Identity: Blockchain provides secure and verifiable digital identity solutions, protecting against fraud and enhancing privacy.
Comparative Analysis of AI and Blockchain Growth
While both AI and blockchain technologies are advancing rapidly, AI's development has been characterized by an accelerated pace and frequent innovations. In contrast, blockchain's growth has been more methodical, focusing on establishing robust, secure systems.
The difference in development speeds can be attributed to the nature of each technology. AI thrives on continuous learning and improvement, driven by data and computational power. Blockchain, however, requires meticulous attention to security and consensus mechanisms, which naturally leads to a slower, more deliberate pace.
Predictions for the Future of AI
Looking ahead, the rapid progression of AI suggests that within the next two years, we will see the emergence of fluent expert assistants capable of taking over real tasks from A to Z. These AI assistants will not only perform routine tasks but also handle complex processes, making informed decisions and providing expert-level support across various domains.
The advancements in natural language processing, machine learning, and AI integration will enable these assistants to operate seamlessly in real-world environments. From managing entire projects to offering personalized advice and support, the capabilities of AI will continue to expand, driving further innovation and transformation across industries.